Public blockchains allow any user to submit transactions which modify the shared state of the network. These transactions are independently verified and executed by a decentralized network of full nodes. Because full nodes have limited computational resources, blockchains limit the total computational resources that can be consumed per unit of time. As user demand may fluctuate, most blockchains implement a transaction fee mechanism in order to allocate finite computational capacity among competing transactions.
The problem
Most existing fee market designs utilize a joint, fungible unit (called gas in Ethereum) to price otherwise non-fungible resources (such as bandwidth, computation, and storage) by hardcoding their relative prices. These one-dimensional fee markets fix the relative price of each resource, which inhibits granular price discovery, opens up the possibility of denial-of-service attacks, and limits scalability as resource needs may change dramatically but the relative prices stay fixed.
To address these issues, many prominent networks such as Ethereum and Solana have proposed multi-dimensional fee markets. In our most recent paper, we provide a principled way to design fee markets that efficiently price multiple non-fungible resources. Importantly, our fee mechanism unifies the competing objectives of the transaction producers (e.g., to maximize their own profit) and of the blockchain designer (e.g., to achieve a target network throughput). Furthermore, our framework provides a precise way to reason about tradeoffs between these competing objectives.
The characters
Blockchain users: Users submit transactions that they want executed on chain. Transactions provide some amount of utility for the user, which may be measurable (for instance, in MEV) or unmeasurable. To ensure their transactions are executed, users must pay fees to the network (for example, the amount burned in EIP-1559) and to the block builders (the tip).
Blockchain validators: Validators run full nodes that verify transactions submitted to the chain. For providing this service, validators are often rewarded by tips, paid by the users, and by rewards from the network. Thus, each transaction that is included provides some utility to the validators, which is a function of the transaction itself, the tips from the transaction, and the computational resources required to validate the transaction.
Blockchain designers: Blockchain network designers aim to maximize the throughput of their network, subject to the hardware specs of the full nodes. The designer sets the full node minimum requirements in a way that balances decentralization and throughput, as lower requirements limit throughput but allow more people to run full nodes for the network. The network designer also may set a resource limit for each block. (For example, in Ethereum, blocks have a gas limit of 30M.) The limit ensures a full node meeting the minimum hardware requirements can reconstruct and verify the current state of the chain (i.e., that full nodes meeting the minimal spec can catch up to the end of the chain in a reasonable amount of time).
In our work, we group the validators and users into one group: the transaction producers. This group has some aggregate utility function of the included transactions and must pay fees to the network in order to include these transactions. Block space markets emerge between the transaction producers, who aim to maximize utility, and the blockchain designers, who aim to maximize the throughput of the network, subject to the minimum hardware requirements.
The mechanism
We start by modeling the resource pricing problem as one in which an omniscient network designer chooses transactions for each block that maximize total welfare subject to demand constraints. Of course, welfare is unknown and likely unmeasurable by the network designer in practice. We show that this problem, which is the ‘ideal end state’ of a blockchain but not immediately useful in itself, decomposes into two problems, coupled by the resource prices. One of these two problems is a simple one which can be easily solved on chain and represents the cost to the network for providing certain resources, while the other is a maximal-utility problem that miners and users implicitly solve when creating and including transactions for a given block. Correctly setting the resource prices aligns incentives such that the resource costs to the network are exactly balanced by the utility gained by the users and miners. This, in turn, leads to block allocations which solve the original ‘ideal’ problem, on average.
The cost of uniform prices
While our paper is mostly theoretical, we also examine a toy model of a blockchain with two resources. We show that pricing resources using our method can increase network efficiency and make the network more robust to DoS attacks or distribution shifts.
Steady-state behavior
We first simulate the steady state behavior of our mechanism with transactions that require roughly equal amounts of resource 1 and resource 2 (e.g., compute and storage).
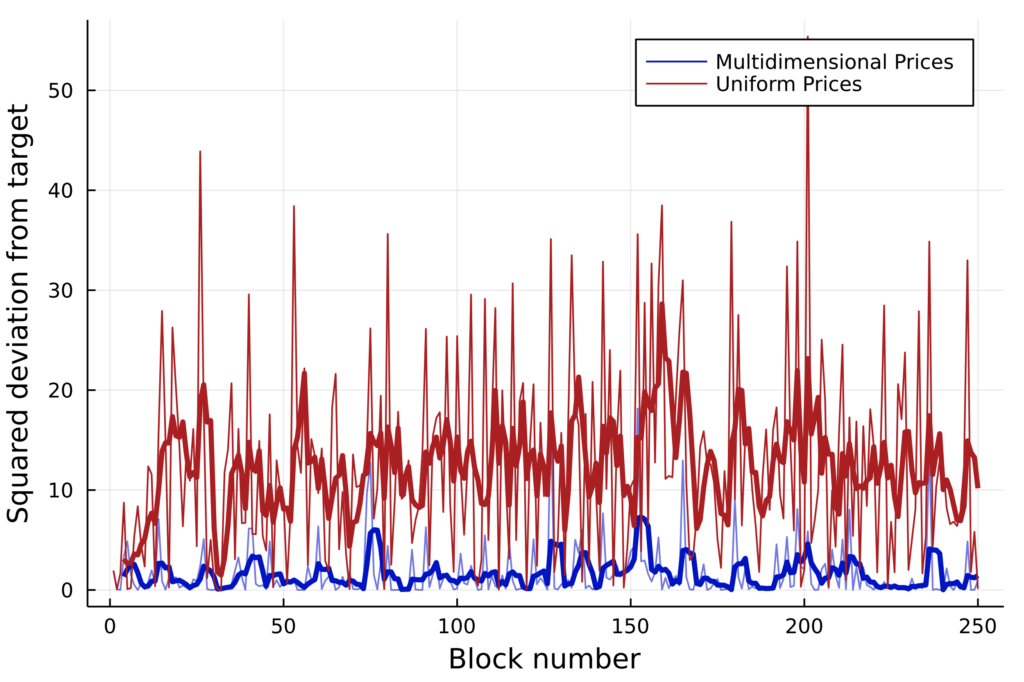
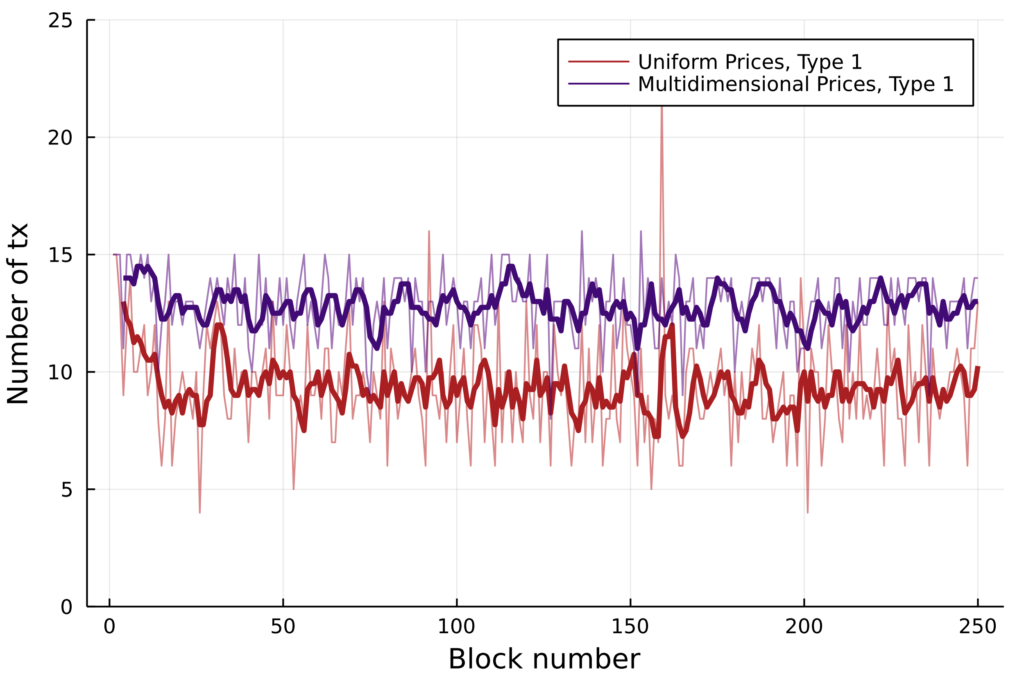
Under steady state behavior, uniform pricing causes higher deviation from resource usage targets (left). This less efficient usage of resources, in the uniform case, also leads to lower throughput (right).
Distribution shifts
We also test mechanism behavior under a distribution shift: we add 150 transactions in block 10 that require a significant amount of resource 2 (e.g., storage). This scenario may appear in an NFT mint, for example.
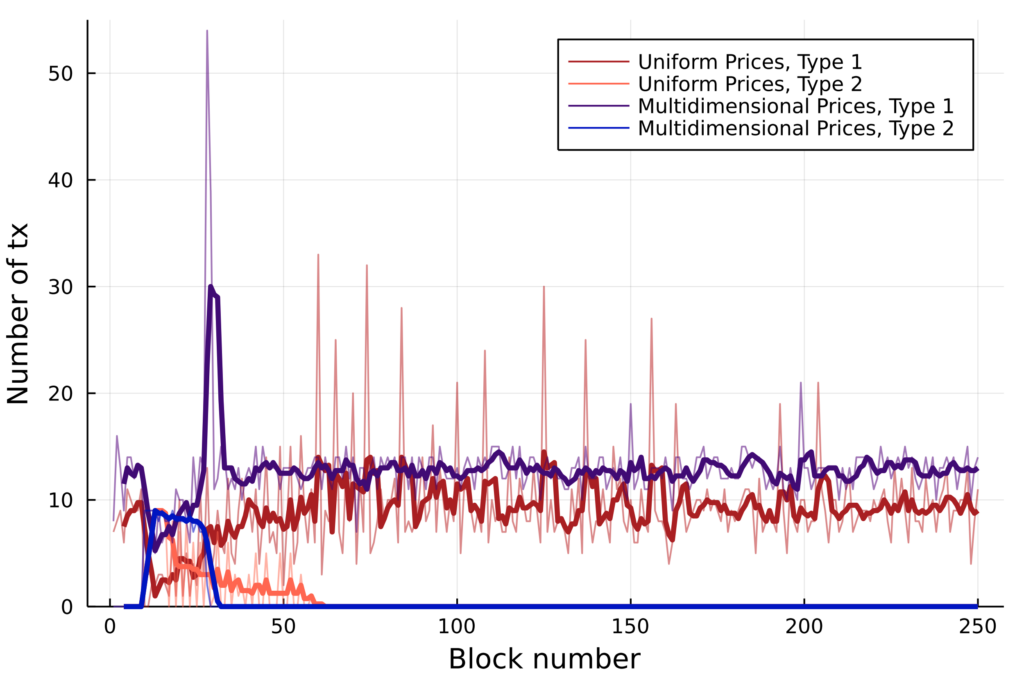
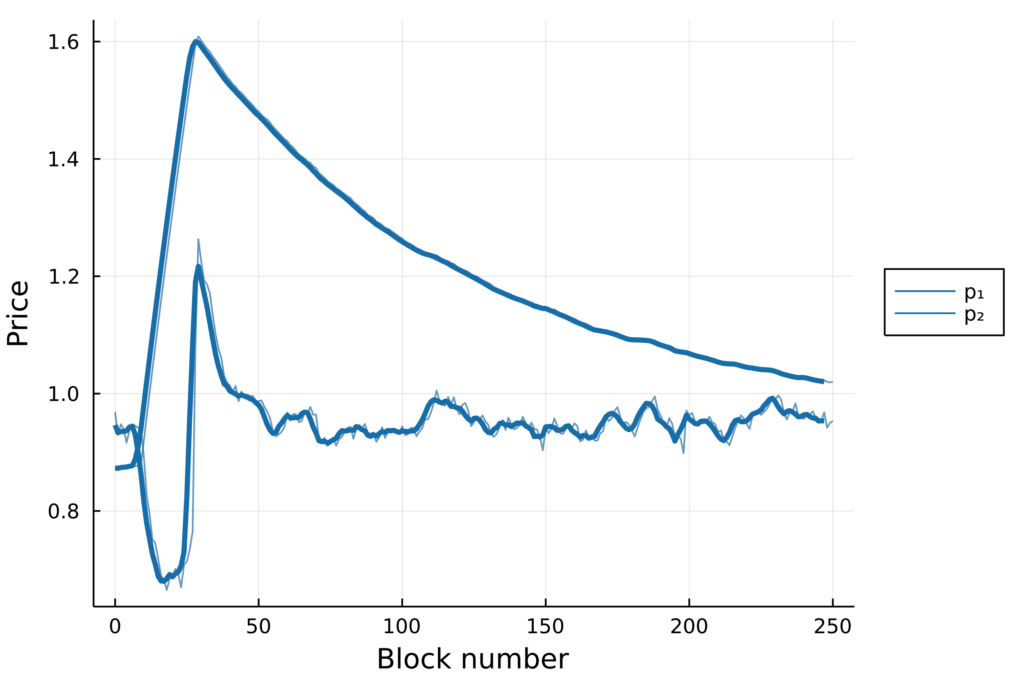
Multidimensional pricing also leads to significantly higher throughput when the distribution shifts (left) by adjusting resource prices accordingly (right).
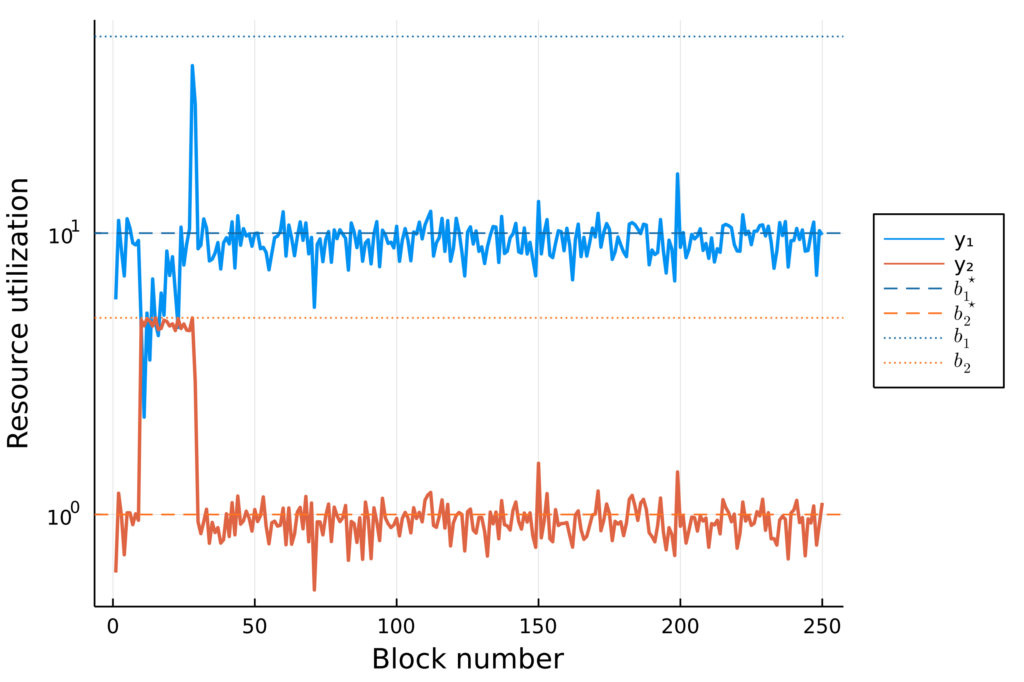
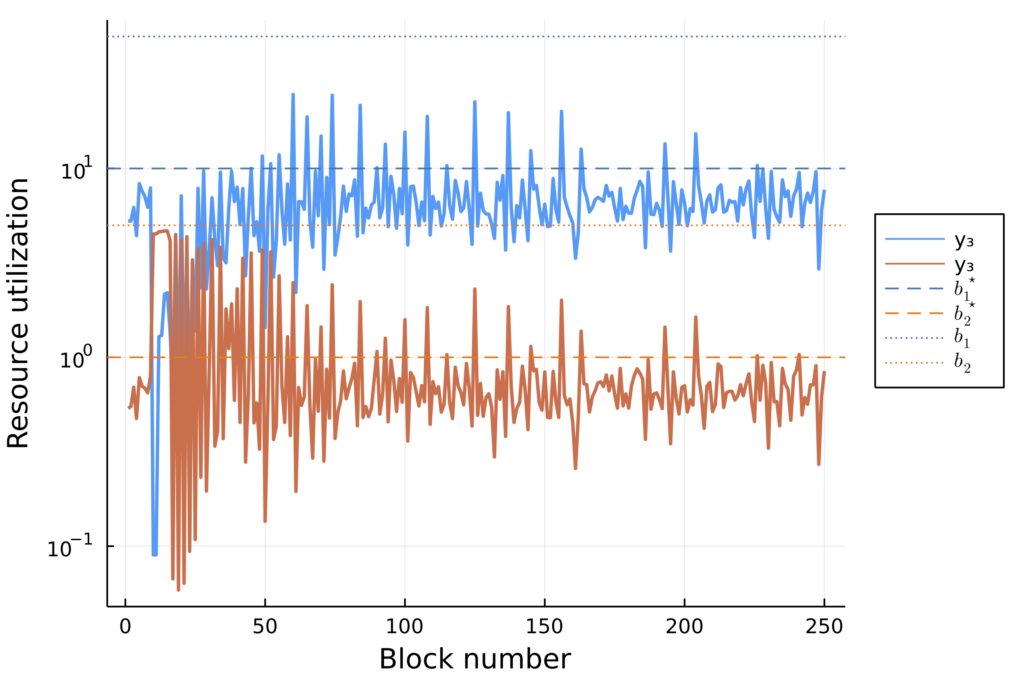
Looking at individual resource usage, we see that multidimensional pricing (left) facilitates smooth, short burst capacity, whereas uniform pricing (right) does not.
Implications and future work
We developed a general framework for multi-dimensional resource pricing in blockchains. While this work is a first step, there’s still plenty of work to do. Future research might include game-theoretic analyses of this mechanism along with more extensive numerical evaluation under realistic conditions. Still, we hope that our work helps base layer blockchain designers, rollup engineers, and application developers optimize fees at all layers of the decentralized stack as they design increasingly complex and performant systems.
For more details, please check out our paper!